13 minutes
Algorithmic Trading in Fintech: Leveraging Machine Learning and High-Frequency Trading
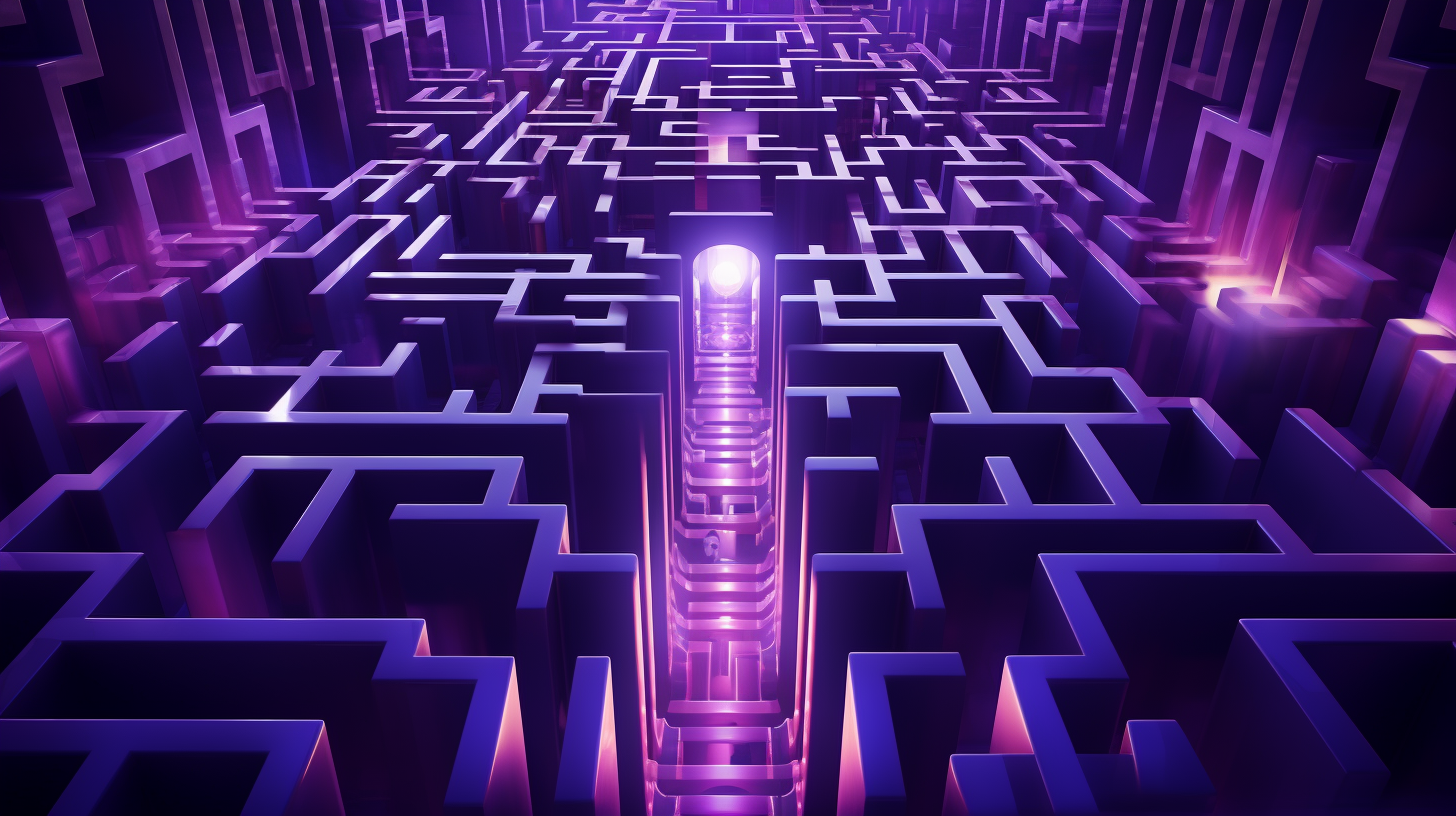
Welcome to the world of algorithmic trading in fintech! In this article, we will explore how algorithmic trading has become a game-changer in the financial industry, thanks to the integration of machine learning and high-frequency trading techniques.
Purple Dash
21/09/2023 7:33 AMTable of Contents
- What is Algorithmic Trading?
- The Role of Fintech in Algorithmic Trading
- Understanding Machine Learning in Algorithmic Trading
- High-Frequency Trading: A Game Changer in Algorithmic Trading
- Risks and Challenges in Algorithmic Trading
- The Regulation and Compliance Landscape in Algorithmic Trading
- The Future of Algorithmic Trading
- Case Studies: Algorithmic Trading Success Stories
- Conclusion
- FAQ
Welcome to the world of algorithmic trading in fintech! In this article, we will explore how algorithmic trading has become a game-changer in the financial industry, thanks to the integration of machine learning and high-frequency trading techniques.
Algorithmic trading is the use of computer programs to execute trades automatically based on pre-set parameters and instructions. The use of algorithms allows financial institutions to analyze vast amounts of market data quickly and make trading decisions faster than humans can.
The rise of fintech has enabled the growth of algorithmic trading by providing advanced technological tools and platforms for creating and implementing automated trading strategies.
Machine learning is one of the most significant innovations in algorithmic trading. It involves the use of historical data and complex algorithms to identify patterns and make predictions based on those patterns. Machine learning algorithms can learn from their own mistakes and adapt to new market conditions, improving trading performance over time.
High-frequency trading, on the other hand, involves the use of advanced technology and algorithms to trade securities at high speeds and volumes, taking advantage of tiny market inefficiencies. HFT has brought increased liquidity and efficiency to financial markets, but it also comes with its own set of risks and challenges.
Key Takeaways:
- Algorithmic trading uses computer programs to execute trades automatically based on pre-set parameters and instructions.
- Fintech has enabled the growth of algorithmic trading by providing advanced technological tools and platforms for creating and implementing automated trading strategies.
- Machine learning involves the use of historical data and complex algorithms to identify patterns and make predictions based on those patterns.
- High-frequency trading involves the use of advanced technology and algorithms to trade securities at high speeds and volumes, taking advantage of tiny market inefficiencies.
- The integration of machine learning and high-frequency trading techniques has revolutionized financial markets, bringing increased efficiency and liquidity, but also new risks and challenges.
What is Algorithmic Trading?
Algorithmic trading, also known as algo trading, is the use of computer algorithms to automate the buying and selling of financial assets. This process differs from traditional manual trading, where humans make all the trading decisions.
In algo trading, the computer executes trades based on a set of predefined rules and criteria. These rules can be developed based on historical data, technical indicators, and other market conditions. By automating the trading process, financial institutions can execute trades faster and more efficiently, with reduced human error and emotions.
Algorithmic trading has become increasingly popular in recent years, with many financial institutions relying on it to gain a competitive edge in the market. The benefits of algo trading include improved speed, accuracy, and efficiency, as well as the ability to analyze large amounts of data in real-time.
The Role of Fintech in Algorithmic Trading
The development of algorithmic trading is largely enabled by advancements in financial technology, or fintech. In recent years, fintech has revolutionized traditional financial markets, providing faster and more efficient solutions to market participants.
The integration of fintech in algorithmic trading has allowed for the utilization of machine learning algorithms in developing trading strategies. Fintech has also facilitated the use of high-frequency trading techniques, enabling market participants to execute trades at lightning-fast speeds.
Moreover, fintech has made algorithmic trading more accessible to a wider range of market participants, including retail traders and small financial institutions. The development of trading platforms and algorithms as a service (AaaS) infrastructure has significantly lowered the entry barrier to algorithmic trading, making it more democratic and inclusive.
Overall, fintech has played a significant role in the growth of algorithmic trading, providing market participants with the tools and infrastructure needed to develop and execute cutting-edge trading strategies.
Understanding Machine Learning in Algorithmic Trading
Algorithmic trading relies heavily on machine learning algorithms to identify patterns, execute trades, and optimize outcomes. Machine learning is a subset of artificial intelligence that allows algorithms to improve automatically through experience, without being explicitly programmed.
Historical data is often used to train algorithms in machine learning, enabling them to recognize patterns and learn from past behavior. This data is often supplemented with real-time market data and news events to refine trading strategies further.
Pattern recognition is a crucial component of machine learning in algorithmic trading. Algorithms can recognize trends in the market, identify anomalies, and predict future outcomes based on similar historical events. These predictions can inform trading decisions, enabling the algorithm to execute trades with greater efficiency and accuracy.
Predictive modeling techniques are also used in algorithmic trading to forecast market movements. These models can recommend trades based on specific criteria, such as changes in market volatility, price trends, or other market indicators. These recommendations can be used to minimize risk and maximize profits.
Applying Machine Learning in Algorithmic Trading
Machine learning algorithms can be applied to various types of financial instruments, including stocks, bonds, currencies, and commodities. These algorithms can analyze vast amounts of data in real-time, enabling institutions to execute trades swiftly and efficiently.
Machine learning algorithms can also be customized to meet specific trading requirements. They can analyze data from various sources, including social media, news outlets, and company reports, to generate insights into market sentiment and future trends.
Moreover, machine learning algorithms can be used to optimize trading strategies continually. They can monitor market conditions, adjust trading parameters, and retrain models accordingly, improving the accuracy and efficiency of trades over time.
In conclusion, machine learning has transformed how algorithmic trading is conducted. Its ability to identify patterns, predict outcomes, and optimize trading strategies has enabled institutions to execute trades more accurately and efficiently, minimizing risk and maximizing returns.
High-Frequency Trading: A Game Changer in Algorithmic Trading
High-frequency trading (HFT) is a type of algorithmic trading that uses sophisticated algorithms to make trades at incredibly high speeds. HFT strategies involve trading large volumes of securities in short time frames, taking advantage of small price discrepancies.
The use of HFT has become increasingly popular in recent years, with many financial institutions using it as a key component of their trading strategies. The advantages of HFT include increased efficiency, lower costs, and the ability to react quickly to market changes.
One of the most common HFT strategies is called "market making." This involves buying and selling securities at bid and ask prices, creating a zero-sum game where profits are made from the bid-ask spread. HFT algorithms are able to perform this strategy at a high frequency, making many trades in a short amount of time.
Another HFT strategy is "arbitrage," which involves taking advantage of temporary price discrepancies in different markets. HFT algorithms are able to detect and exploit these discrepancies in milliseconds, generating profits for market participants.
However, HFT has also faced criticism, with some arguing that it creates market instability and makes it difficult for traditional long-term investors to compete. There have also been concerns around the potential for market manipulation and the risks of system failures.
Technology Involved in High-Frequency Trading
HFT relies on advanced technology to execute trades at incredible speeds. This includes the use of co-located servers that are placed as close as possible to stock exchange servers to reduce latency. HFT algorithms also use high-speed data connections and advanced data analysis techniques to make trading decisions in real-time.
The hardware used in HFT includes field-programmable gate arrays (FPGAs) and application-specific integrated circuits (ASICs) that are designed specifically for trading-related computations. These specialized chips are able to process data at incredibly high speeds, giving HFT algorithms a significant advantage over traditional trading methods.
The use of HFT is likely to continue to grow in the coming years, as technological advancements allow for even faster trading speeds and more sophisticated trading strategies.
Risks and Challenges in Algorithmic Trading
While algorithmic trading offers numerous benefits to financial institutions, it also presents several potential risks and challenges.
Market Volatility: One of the major risks associated with algorithmic trading is its susceptibility to market volatility. Automated trading systems are designed to respond quickly to market fluctuations, but in extreme market conditions, they may amplify price movements, leading to increased volatility.
System Failures: Another significant risk is the potential for system failures. Glitches, bugs, or connectivity issues can result in unintended trades or complete shutdowns of automated trading systems, causing substantial financial losses.
Market Manipulation: Algorithmic trading can also be exploited for market manipulation purposes. For example, traders can use the systems to execute trades that influence market prices, leading to illegitimate gains or losses for others.
Regulatory Concerns: Finally, there are regulatory concerns associated with algorithmic trading. Regulators are concerned about the potential for market abuse, and they have implemented rules and guidelines to ensure fair and orderly markets. As a result, firms engaging in algorithmic trading must comply with stringent rules and reporting requirements.
Overall, financial institutions must carefully manage the risks associated with algorithmic trading to ensure its continued success and avoid potential regulatory and reputational damage.
Conclusion:
In conclusion, algorithmic trading has revolutionized financial markets, enabling financial institutions to execute trades at lightning speeds and with increased accuracy. However, the use of automated trading systems also presents several potential risks and challenges that must be carefully managed. With appropriate risk management strategies in place, algorithmic trading can continue to play a crucial role in the future of finance.
The Regulation and Compliance Landscape in Algorithmic Trading
The rise of algorithmic trading has triggered concern among regulators over the potential risks it poses to financial stability. Despite the advantages that automated trading systems offer, they have been implicated in several high-profile market disruptions, including the flash crash of 2010 and the Knight Capital Group trading glitch of 2012. In response, regulators have implemented various measures aimed at ensuring fair and orderly markets while promoting innovation and competition.
The Regulatory Landscape
The regulatory landscape surrounding algorithmic trading is multifaceted, with several governing bodies involved. In the US, the Securities and Exchange Commission (SEC) and the Commodity Futures Trading Commission (CFTC) have primary jurisdiction over securities and derivatives markets, respectively. Both agencies have implemented rules targeting algorithmic trading, including risk controls, market access requirements, and reporting obligations.
Similarly, the European Union has enacted a comprehensive set of regulations governing algorithmic trading, known as the Markets in Financial Instruments Directive (MiFID II). The directive imposes strict requirements on market participants, including pre-trade risk assessments, order-to-trade ratios, and post-trade surveillance obligations. MiFID II also mandates the use of standardized messaging protocols to facilitate communication between trading venues.
Compliance Requirements
Market participants in algorithmic trading are subject to several compliance requirements aimed at promoting market integrity and mitigating potential risks. These include:
- Pre-trade risk controls: Trading algorithms must be subject to pre-trade risk limits to prevent orders that could create undue risk to the market.
- Market access controls: Firms must implement measures to prevent unauthorized access to trading systems.
- Post-trade surveillance: Firms must monitor market activity for potential manipulation or other abusive practices.
- Reporting obligations: Firms must report certain order and trade data to regulators to ensure transparency and market oversight.
Non-compliance with these requirements can result in significant penalties and reputational damage for firms, underscoring the importance of robust compliance programs.
"The rise of algorithmic trading has triggered concern among regulators over the potential risks it poses to financial stability."
The Way Forward
As algorithmic trading continues to evolve, regulators will need to remain vigilant in ensuring fair and orderly markets while promoting innovation and competition. The use of machine learning algorithms and other advanced technologies in trading systems will require ongoing monitoring and assessment to identify potential risks and vulnerabilities.
However, with proper risk management and compliance protocols in place, algorithmic trading has the potential to enhance market efficiency and liquidity while reducing costs for market participants. As such, it is essential for regulators and industry participants to work collaboratively to strike a balance between innovation and market integrity.
The Future of Algorithmic Trading
Algorithmic trading has come a long way since its inception, and the future looks even brighter. With the rapid advancement of technology, the potential for innovation is limitless. Here are some of the emerging trends in algorithmic trading:
Reinforcement Learning
Reinforcement learning is a subfield of machine learning that focuses on training artificial agents to make decisions based on the actions that yield the highest reward. It has shown great promise in the context of algorithmic trading, as it can help to identify profitable trading strategies in complex market conditions.
Natural Language Processing
Natural language processing (NLP) is a technology that enables computers to understand and interpret human language. In the context of algorithmic trading, NLP can be used to analyze news articles, social media posts, and other sources of market data to identify patterns and sentiment that may influence trading decisions.
Quantum Computing
Quantum computing is a cutting-edge technology that has the potential to revolutionize algorithmic trading. Quantum computers can perform complex calculations at lightning speed and offer the potential to develop more sophisticated trading algorithms than ever before.
As the world of algorithmic trading continues to evolve, it's important to stay up-to-date on the latest trends and developments. By leveraging the power of machine learning, high-frequency trading, and other advanced technologies, financial institutions can gain a competitive edge in the market and generate substantial profits. However, it's important to remain vigilant of potential risks and challenges associated with automated trading systems and stay compliant with regulatory requirements.
Case Studies: Algorithmic Trading Success Stories
Real-world examples have shown the overwhelming potential of algorithmic trading. Let's dive into a few case studies that highlight its success.
Quantitative Investment Management
"Quantitative investment management has been using algorithmic trading for over two decades, and their performance speaks for itself. They have generated an average annual return of 20% since 1988, far outpacing the S&P 500 Index."
Quantitative Investment Management is a hedge fund that uses computerized trading models based on quantitative analysis to make investment decisions. They use a variety of algorithms, including trend following, mean reversion, and statistical arbitrage.
Renaissance Technologies
Renaissance Technologies is another hedge fund that has leveraged algorithmic trading to great success. Their flagship fund, the Medallion Fund, has generated average annual returns of 66% since 1988.
The team at Renaissance Technologies uses a combination of machine learning algorithms and statistical models to identify market inefficiencies and make profitable trades. They analyze vast amounts of historical data to identify patterns and trends that are not visible to the naked eye.
JP Morgan Chase
JP Morgan Chase is one of the largest financial institutions in the world, and they use algorithmic trading to improve efficiency and reduce risk.
One of their most successful algorithmic trading systems is LOXM, which uses machine learning algorithms to trade equities. LOXM has generated an average annual return of 14% since its inception in 2012.
JP Morgan Chase also uses algorithmic trading to execute trades quickly and efficiently. By leveraging high-frequency trading techniques, they are able to execute trades in milliseconds, giving them a competitive advantage in the market.
Conclusion
These case studies highlight the immense potential of algorithmic trading in generating profits for financial institutions. By leveraging machine learning and high-frequency trading techniques, firms can identify and capitalize on market inefficiencies that would be impossible to detect manually.
However, it is important to keep in mind the potential risks and challenges associated with algorithmic trading, including system failures, market manipulation, and regulatory concerns. As such, it is crucial for market participants to implement strong risk management and compliance protocols.
Conclusion
In conclusion, algorithmic trading in fintech has completely transformed financial markets. With the integration of machine learning algorithms and high-frequency trading techniques, financial institutions now have the ability to analyze vast amounts of data, identify market trends, and execute trades at lightning speed.
While algorithmic trading offers numerous benefits, it also comes with potential risks and challenges, such as system failures, market manipulation, and regulatory concerns. As such, it is crucial for market participants to stay up-to-date with regulatory requirements and compliance standards.
Looking ahead, algorithmic trading is poised to continue evolving with emerging trends such as reinforcement learning and natural language processing. The potential impact of quantum computing on trading strategies also holds exciting possibilities for the future.
Case Studies: Algorithmic Trading Success Stories
Real-world examples of successful algorithmic trading strategies have demonstrated the potential for substantial profits in financial markets. One such example is Renaissance Technologies, a hedge fund that has consistently outperformed the market using quantitative trading strategies.
Another success story is Two Sigma Investments, which uses machine learning algorithms to inform its trading decisions. The firm's flagship fund, the Two Sigma Absolute Return Fund, has consistently generated positive returns since its inception.
These successful implementations of algorithmic trading showcase the potential for financial institutions to generate substantial profits while also improving market efficiency. As technology continues to advance, the possibilities for algorithmic trading are endless.
As the financial landscape continues to evolve, algorithmic trading will undoubtedly play a significant role in shaping the future of financial markets. It is an exciting time for fintech and algorithmic trading, and we look forward to seeing what the future holds.
FAQ
Q: What is algorithmic trading?
A: Algorithmic trading is a method of executing trades in financial markets using pre-programmed instructions. It involves the use of automated systems that analyze market data, identify trading opportunities, and execute orders without human intervention.
Q: How does algorithmic trading differ from manual trading?
A: Algorithmic trading differs from manual trading in that it relies on computer programs and algorithms to make trading decisions. Manual trading involves traders making decisions based on their own analysis and intuition.
Q: What are the benefits of algorithmic trading?
A: Algorithmic trading offers several benefits, including increased execution speed, improved accuracy, and the ability to execute trades at a large scale. It also eliminates human emotion and bias from the trading process, leading to better decision-making.
Q: What is the role of fintech in algorithmic trading?
A: Fintech plays a crucial role in algorithmic trading. It provides the technology and infrastructure necessary for the development and implementation of automated trading systems. Fintech has enabled faster and more efficient execution of trades, as well as the integration of machine learning algorithms for improved performance.
Q: How is machine learning applied in algorithmic trading?
A: Machine learning is applied in algorithmic trading to analyze large volumes of historical data, identify patterns and trends, and make predictions about future market movements. It allows trading algorithms to adapt and improve over time, leading to more accurate and profitable trading strategies.
Q: What is high-frequency trading (HFT) and how does it impact algorithmic trading?
A: High-frequency trading (HFT) is a type of algorithmic trading that involves executing a large number of trades at extremely high speeds. HFT strategies rely on advanced technology and powerful computers to take advantage of small price discrepancies in the market. HFT has significantly increased market liquidity and efficiency, but it has also raised concerns about market manipulation.
Q: What are the risks and challenges associated with algorithmic trading?
A: Algorithmic trading comes with its share of risks and challenges. System failures, such as software glitches or connectivity issues, can lead to substantial financial losses. There is also the risk of market manipulation or algorithmic errors causing large-scale disruptions. Regulatory concerns and compliance requirements add another layer of complexity to algorithmic trading.
Q: How is algorithmic trading regulated?
A: Regulatory bodies have implemented measures to ensure fair and orderly markets in algorithmic trading. These measures include requirements for risk controls, such as circuit breakers, to prevent excessive market volatility. Market participants are also required to comply with specific reporting and disclosure obligations to ensure transparency and accountability.
Q: What does the future hold for algorithmic trading?
A: The future of algorithmic trading looks promising. Emerging trends such as reinforcement learning and natural language processing have the potential to further enhance trading strategies. Additionally, the development of quantum computing may revolutionize algorithmic trading by enabling more complex and efficient calculations.
Q: Can you provide any real-world examples of successful algorithmic trading strategies?
A: There have been several successful algorithmic trading strategies in the financial industry. For example, trend-following strategies that aim to capitalize on long-term market trends have generated substantial profits. Statistical arbitrage strategies that exploit pricing anomalies between related securities have also been successful. Each case study provides valuable insights into the implementation and effectiveness of algorithmic trading strategies.
Latest Articles
View AllStay up-to-date with the latest industry trends and insights by reading articles from our technical experts, providing expertise on cutting-edge technologies in the crypto and fintech space.